May 2025 | Volume 26 No. 2
Cover Story
Modelling Human Vision
In every waking moment, our eyes take in an enormous amount of information from our environment – people, places, things, animals, events. But how does our brain identify and organise these images, and single out the important ones?
That question is of interest to both neuroscientists, who want to better understand the workings of the brain, and computer scientists, who want to apply that to computer vision. Professor Andrew Luo, Assistant Professor in the HKU Musketeers Foundation Institute of Data Science and Department of Psychology, comes from both backgrounds and he has produced insights that enrich our knowledge about visual processing.
“I study a region of the brain called the visual cortex, which processes everything we see. To study this in the past, you would have had to recruit graduate students and do a lot of experiments to show images to human subjects and record their brain activity. But now, we can do data-driven studies using tools like generative image diffusion models and large language models in a way that is accelerating scientific discovery,” he said.
His own work uses functional magnetic resonance imaging (fMRI) to record the brain as it responds to thousands of random images. This is then fed into computer tools, such as image diffusion models and large language models, to identify semantic trends and understand which kinds of images activate which areas of the brain.
Survival needs
A key finding is that the organisation of the visual cortex corresponds to human evolutionary needs, with one component responding to bodies and faces, another to place areas and physical scenes, and a third component to food.
“To survive, a person needs to recognise friends and family, they need to know where they need to go, and they need to find food. This is an exciting observation because it finds that the visual cortex is strongly ecologically driven by these survival needs,” he said.
The results have enabled him to stimulate brain activity in specific ways by generating images with different attributes. They have also enabled him to develop computer tools based around the brain’s image processing.
One tool he developed, called BrainDIVE (Brain Diffusion for Visual Exploration), creates images predicted to activate specific regions of the brain, having been trained on a dataset of natural images paired with fMRI recordings.
This bypasses the need to hand-craft visual stimuli. Another tool, BrainSCUBA (Semantic Captioning Using Brain Alignments), generates natural language captions for images, which can in turn be used to generate new images. Finally, a tool called BrainSAIL (Semantic Attribution and Image Localization) allows for the disentanglement of complex natural images.
The combination of image and language builds on findings from the past decade that show vision models are best able to predict the areas of the brain activated by certain images when they are ‘self-supervised’ and left to their own devices. For example, such models are better at distinguishing between images of a dog and a cat if they can figure it out themselves rather than be told what to look for in a dog and a cat.
More robust
They are also better at semantic coherence, meaning they can understand when objects are related even if their individual components seem a little off. For instance, the red colour of pepperoni on pizza could lead models based on visual similarity, without the semantics component, to conclude the pizza was uncooked.
“Semantically self-supervised models are more robust to visual dissimilarity in the world because of how they classify objects,” Professor Luo said. “They can learn more flexible representations, potentially in a more similar way to humans.”
Vision is not the only input that could generate such outcomes. Professor Luo has also been trying to integrate sound into generative models to improve their representations. But vision is still his key focus, given it is the most dominant of the human senses. He hopes to explore the hierarchy of perception and apply his findings to the brain-computer interface.
“I came to HKU in last September and it’s a great place because there are a lot of people doing cross-disciplinary work in data science or machine learning combined with another field.
“Going forward, I hope to develop better tools to understand the human brain and to use the human brain to interact with, for example, brain-controlled robotics. I also want to leverage insights from human cognition and psychology to design better models,” he said.
Semantically self-supervised models are more robust to visual dissimilarity in the world because of how they classify objects. They can learn more flexible representations, potentially in a more similar way to humans.
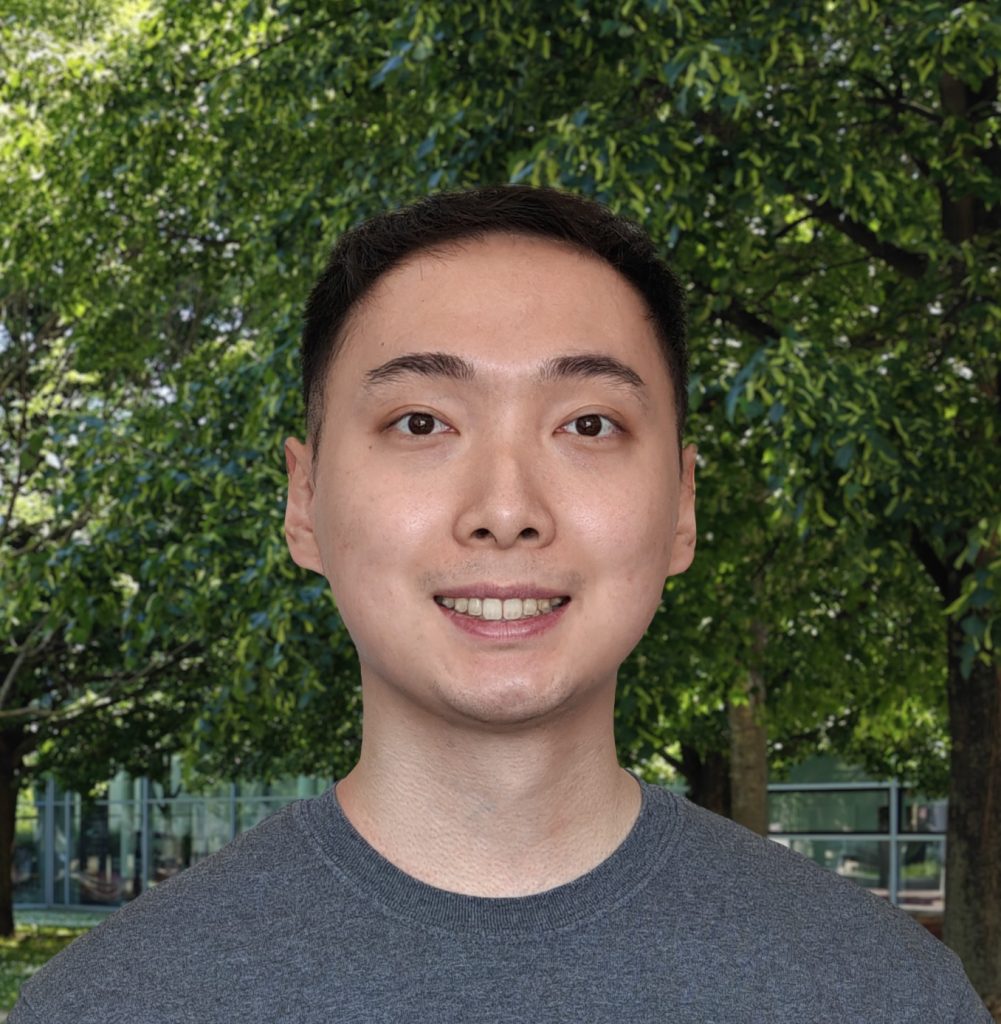
Professor Andrew Luo