May 2025 | Volume 26 No. 2
Cover Story
The Challenges with Computer Vision
The power of computers to ‘see’ – to recognise visual patterns – is being tapped for all sorts of purposes, from enabling autonomous vehicles, to providing fast and comprehensive analysis of medical image results, to recognising faces. But there are inherent traps that can undermine trust in the technology.
Bias and discrimination, liability issues, and corporate surveillance are all areas where computer vision has raised red flags, says Professor Boris Babic, joint Associate Professor at the HKU Musketeers Foundation Institute of Data Science, Department of Philosophy, and Faculty of Law. And while some of these issues have been widely acknowledged, others are not receiving sufficient attention.
Much of the focus in popular and academic literature has been on the bias problem. For instance, computer vision reportedly has difficulty distinguishing between faces of black people and between men and women. The problem is likely down to the training data used, but there are real-world consequences.
“One obvious issue is that if we apply computer vision systems to public policy and criminal procedure – basically any sensitive decision-making context that requires allocating scarce resources or imposing costs like criminal punishment – then there is going to be a concern about making sure the systems are performing roughly equally among all relevant subgroups,” he said.
However, this may be a bigger problem in societies with a history of racial segregation, such as the US, since discrimination and bias may already be embedded in the data used to train computer vision systems there.
Corporate surveillance concerns
A wider issue is liability, particularly in the case of autonomous vehicles. While manufacturers could be held accountable, what happens when an accident is triggered through shared data? Think in terms of something like the digital navigator Amap in China, which is used by the automaker BYD, or an equivalent in other countries.
“If every company is developing its own AI system and its own data, and everything is closed to others, maybe it’s easy to determine liability. But how do you re-conceive responsibility when you have multiple subsystems contributing to a decision in a way that’s going to make it increasingly hard to decipher which part of the decision belongs to which subsystem?” Professor Babic said.
Some car companies have started experimenting with insurance coverage for autonomous cars, but the question probably will not be settled until there are compelling test cases in court.
In any case, the collection of data by companies raises the problem of corporate surveillance. Carmakers and many other companies, such as phone manufacturers and online browsers, collect user data ostensibly for functionality. But what those companies can then do with the data is not really addressed by privacy protection laws, he said. For example, should insurance companies be prevented from using a driver’s recorded driving speed to set their insurance premiums? If yes, what assurance can there be that the data is not shared with adjusters in the company? “I think corporate surveillance and espionage get way too little attention. Most of the attention is on government or police surveillance, which is low-hanging fruit because their cameras are often conspicuous and you can visit government contract websites to see what they are ordering.
“Whereas with corporate data, we have no idea how their models are updated or what happens with a lot of this data, or how it’s combined with other data sources. If you have a self-driving car with radar and imaging systems, they can track your every movement, but it’s not clear where the data is going,” he said.
Explainability might not improve things
Other worrying examples of corporate use are medical scans and other health data, which, if accessed by insurers, could affect a person’s premiums.
New laws or legal approaches might potentially address liability and surveillance. But when it comes to bias and fairness, the response has been driven by engineers: open the black box of AI and try to fix the problem there. Intriguingly, Professor Babic is not in favour of this approach.
“Computer vision is a paradigm example of a black box system because it is high-dimensional and the features are not intuitive. There has been a large area of research on how to make these systems explainable or transparent, but I think we should accept they are doing something quite different from how our own brains process and recognise images. Rather than attempt to make them understandable to us, we should instead focus on their performance. Because explainability does not necessarily make performance better,” he said.
Accepting that the technology operates differently from humans could also help sharpen focus and resources on the important issues of liability, whether decisions are autonomous or informed by human judgement, and how the data is used. “We should be looking at what it is doing and whether it is improving decision-making in society for whatever context we’re appropriating it for,” he added.
If we apply computer vision systems to public policy and criminal procedure, then there is going to be a concern about making sure the systems are performing roughly equally among all relevant subgroups.
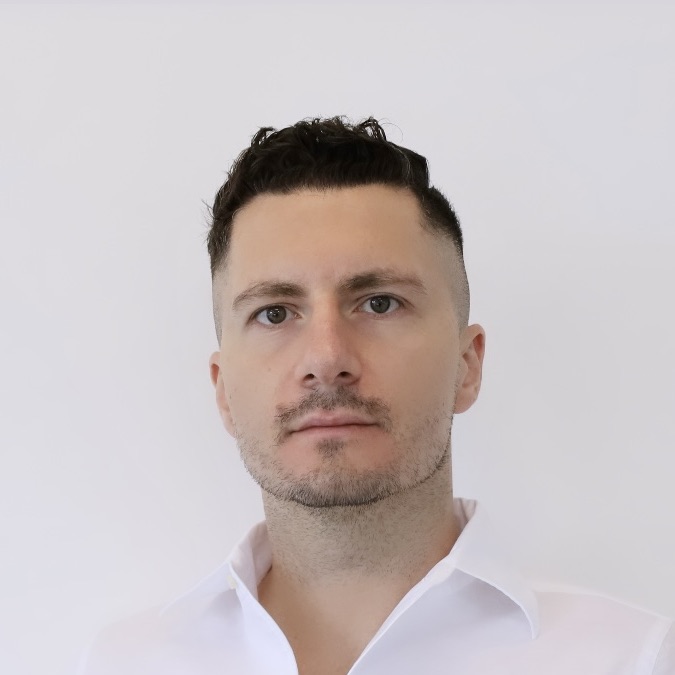
Professor Boris Babic