November 2020 | Volume 22 No. 1
Facial Recognition: The Next Phase
A decade ago, facial recognition technology that used artificial intelligence (AI) and computer vision was still largely confined to the laboratory. That was about to change and among the pioneers was Dr Ping Luo of the Department of Computer Science.
Dr Luo was doing his PhD at the time and his supervisors suggested that he apply deep learning to improve the accuracy of facial recognition. The outcome of his work included a facial recognition system that in 2014 outperformed submissions by Google, Facebook and others in the gold standard for human vision performance, the Labelled Faces in the Wild test.
“With conventional methods, you need to define the feature manually, such as the contours or boundaries of the face. With deep learning, it’s an end-to-end model that uses the raw data, which are the pixels, to automatically learn facial features. It discovers how to distinguish and represent a human face,” he said.
The system he helped develop, called Deep ID, was based on a unique dataset that he also helped compile, called CelebA. The latter has become the most widely used image database in the world for training machines to recognise faces.
CelebA is short for Celebrity Attribute Dataset and it is based on about 200,000 images of celebrities that were collected from the Internet. Human researchers labelled attributes such as hair styles, eye and mouth shapes, and expressions such as smiling, and this information was fed into Deep ID.
Better than humans
CelebA has been cited more than 2,700 times by other AI researchers, but there is a downside: it has also been used to generate fake images that are so realistic, even humans cannot tell they are fake. “Unfortunately, there are many fake videos based on this technology. So we are also doing research on a system that would detect if an image is fake or real,” Dr Luo said.
The facial recognition system, meanwhile, has been picked up by government clients, metro operators and retailers in Mainland China through the start-up SenseTime (founded by one of his PhD supervisors, Professor Tang Xiaoou), where Dr Luo worked for five years before joining HKU in 2019. It has been used for such things as enabling people to use the metro or pick up items from shops based on facial recognition, without the need for money or a card.
Dr Luo acknowledges people may have privacy concerns, but points out that facial recognition is already part of everyday life around the world through smartphones. Apple phones, for instance, have facial ID. “Facial recognition application is very active in mobile computing and it can also be useful for other purposes,” he said.
Apart from recognising faces, Dr Luo has also completed a General Research Fund (GRF) project to develop technology that analyses social relations between people, such as whether they are friendly or competitive, based on facial expressions. This could help people who have difficulty understanding human emotions and assist in the development of robots that are better at interacting with humans.
“With Deep ID and CelebA, we focussed on a single human face. Here we have been looking at both photos and videos. Our proposed algorithm was state of the art and we want to invite others to improve its accuracy,” he said.
Exact match
Dr Luo has also applied AI to fashion, developing the DeepFashion benchmark that can help users find clothes online based on a single image, such as a photo of a celebrity wearing a desired pair of jeans. The matching accuracy is very high.
“We consider each piece of clothing as an identity. Just as we identify the human face, we can assign an identity to a single image of clothing,” he said. “This is much more difficult than labelling a human face, which has a regular structure and components.”
The database underpinning DeepFashion includes more than one million images of clothes, which were labelled by more than 500 people over three months. Key ‘landmarks’, such as colour or shoulder type, were applied to each image. In the first phase, only a maximum eight landmarks were identified but the recent second phase has up to 50 landmarks per clothing item. The aim is to have an exact match, not a ‘similar’ item.
Moving forward, Dr Luo is interested in exploring the theory of deep learning and extending its application to other areas, particularly reinforcement learning which is used to build decision-making systems in robots. Some of his students are also investigating its use in self-drive cars.
These achievements led to Dr Luo being selected one of 20 Innovators Under 35 in the Asia Pacific region this year by MIT Technology Review. “It was a big surprise for me,” he said.
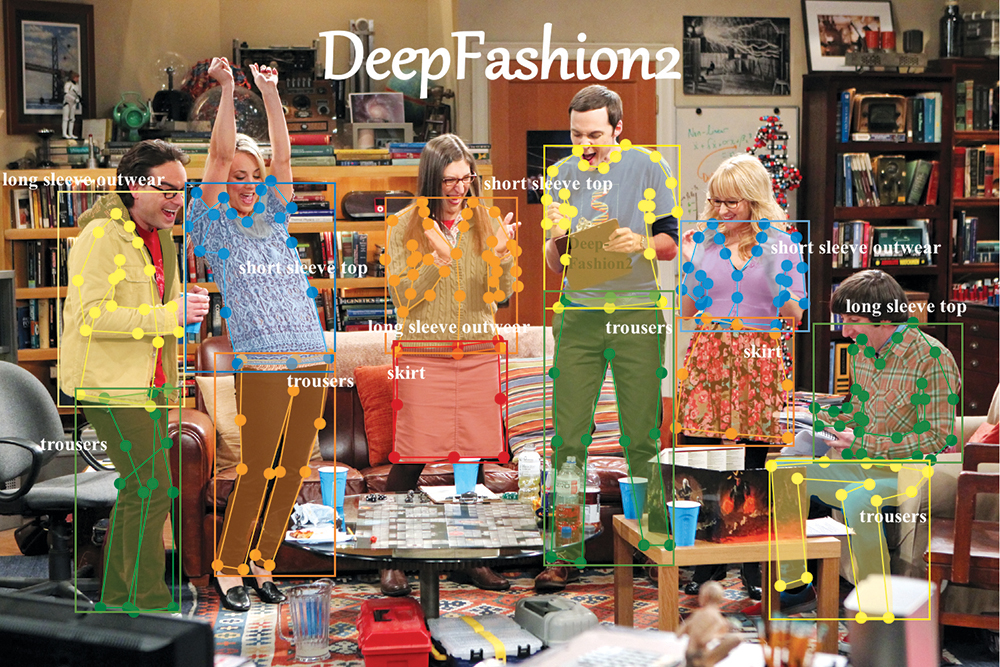
DeepFashion2, an advanced version of DeepFashion, is a large scale benchmark of fashion images, enabling a full spectrum of tasks of fashion image understanding such as fashion retrieval, landmark detection, virtual try-on.
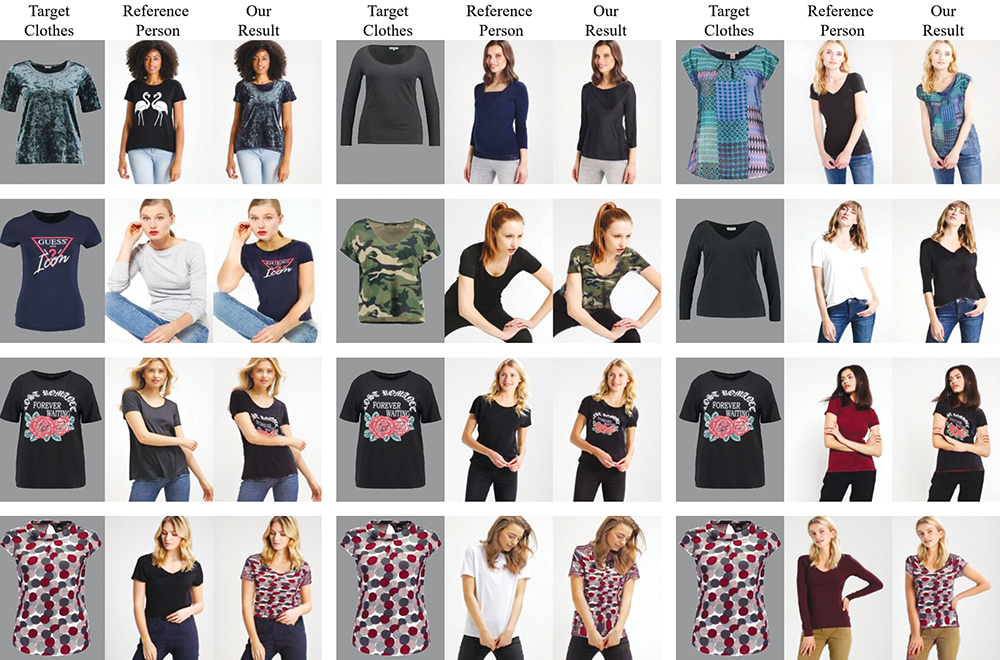
A virtual try-on system has been built using the DeepFashion2 data. These results may enable hyper personalisation fashion design and production in the future.
Unfortunately, there are many fake videos based on this technology. So we are also doing research on a system that would detect if an image is fake or real.
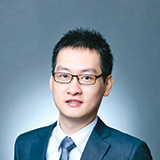
DR PING LUO