May 2025 | Volume 26 No. 2
Cover Story
Computers Take the Wheel
Listen to this article:
Self-driving cars are no longer a vision of the future. They are already operating in some streets. Google’s Waymo, which develops self-driving vehicles, is up and running in several US cities and this year started testing in Japan. Goldman Sachs predicted in August 2024 that partially autonomous cars would comprise 10 per cent of new vehicle sales by 2030.
Handing the wheel over to a machine might seem risky, but it has been made feasible thanks to advances in computer vision and the development of new AI-based systems to process visual data, such as that developed by Professor Li Hongyang, Assistant Professor in the HKU Musketeers Foundation Institute of Data Science and a member of OpenDriveLab.
In 2023, Professor Li and his team unveiled Unified Autonomous Driving (UniAD), which combines different modules, such as image recognition and action planning, into one end-to-end network. Usually, these modules function separately, but when combined, they are much more effective and accurate at processing visual signals.
The research won the best paper award at the prestigious 2023 IEEE/CVF Conference on Computer Vision and Pattern Recognition and its end-to-end pipeline approach has since been widely adopted by several renowned automakers globally, generating a wide spectrum of customised versions of end-to-end driving solutions in various scenarios.
Adopted by carmakers
“We are the originators of the technical roadmap for end-to-end pipeline technology, which has provided the industry with a prototype verification to follow up on,” Professor Li said.
“Private companies will never say that they have adopted methods developed in academia, but Tesla now uses this kind of technology. So does Waymo and a number of car manufacturers in Mainland China, such as Huawei and Xiaomi.” In fact, he is collaborating with such a manufacturer to test the latest version of UniAD in vehicles using the platform World Engine.
In the traditional approach, there is a multi-step process where the camera takes images that are interpreted based on manually-input rules, the planning module then decides the trajectory of the vehicle, and the execution module takes the appropriate action. With UniAD, this is all integrated into one flow of action by using AI to train the model in recognition and response, and to optimise features, without any explicit design or intermediary.
The team did a detailed comparison between UniAD and conventional systems and found that the relative gain or improvement in various tasks ranged from 20 per cent to 100 per cent, depending on the task.
Eyes on robotics
Despite that, though, Professor Li said there are still obstacles to the widespread use of autonomous vehicles, in particular regulatory and liability issues. His team and collaborators have therefore been looking at how to apply their know-how in another kind of system – robotics. Autonomous vehicles and robotics are similar in that they both involve movable rigid objects that can embody AI.
“As AI advances and is able to recognise objects, the next step is to physically interact with the environment. Robotics naturally fit in this category, so we are working on topics like humanoids and manipulation, including our new AgiBot World platform, which is a large-scale dataset for robotic manipulation,” he said.
A recent project is training a robotic arm to recognise objects on a table and tidy them up in an organised way, similar to how humans would perform the task, rather than simply grabbing at the objects. This is not yet the humanoid robot that many envision could be a playmate or personal maid, but it is a necessary step towards developing an intelligent robotic system for AGI 2.0 – the next generation of artificial general intelligence.
“Robotics is still at a very early, preliminary stage. Robots can only move something from one place to another from a fixed position. The key challenge is in making robots mobile and able to perform tasks like a human would. You have to train them with a large amount of data that is very diverse and challenging, and it may be 5 or 10 years away before we can do this. But there are a lot of researchers and start-ups working on it,” he said.
That includes Professor Li and his team, who hope that their approach of using computer vision and an end-to-end pipeline will yield new discoveries in the field.
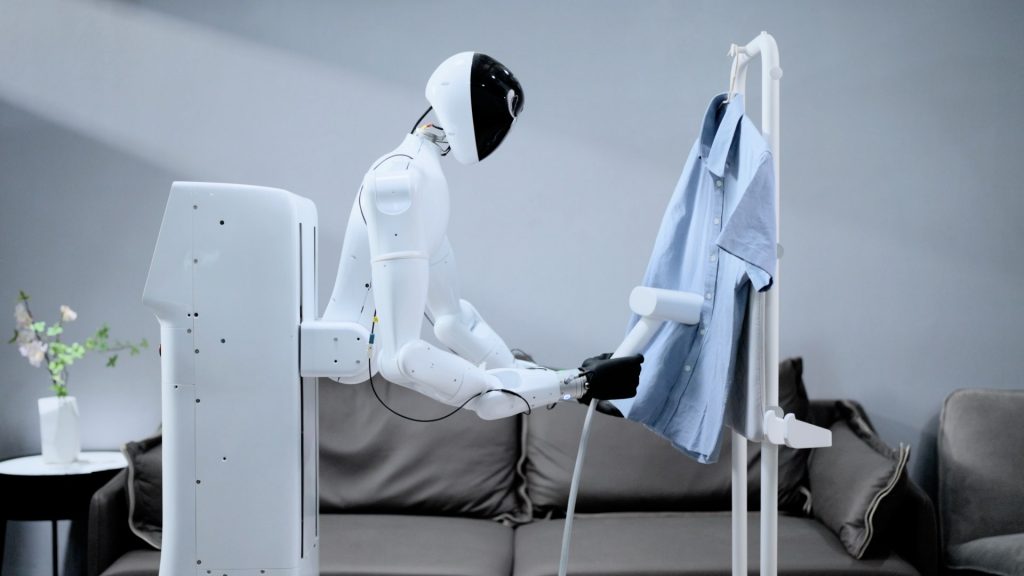
Picture of a robot performing a household task from OpenDriveLab’s AgiBot World, an open-source platform to advance humanoid robot training and development.
We are the originators of the technical roadmap for end-to-end pipeline technology, which has provided the industry with a prototype verification to follow up on.
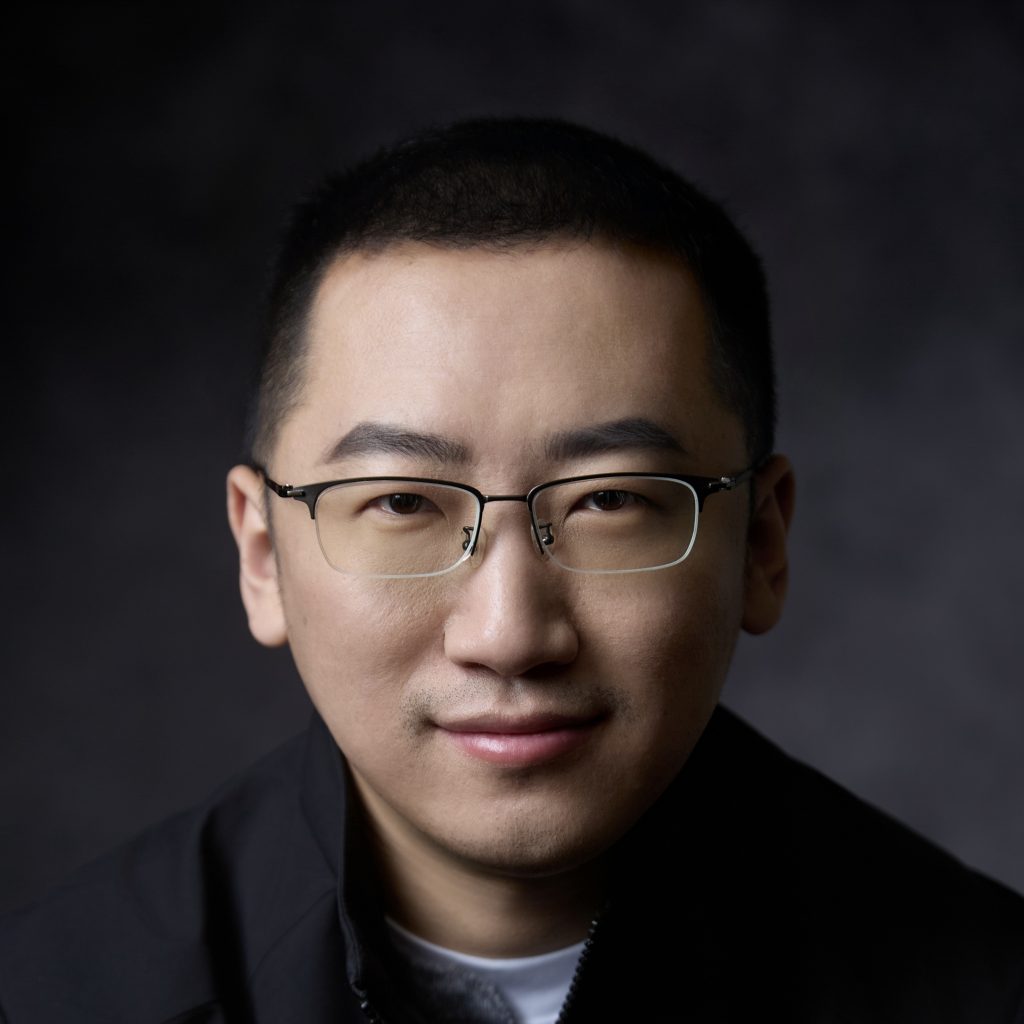
Professor Li Hongyang