May 2025 | Volume 26 No. 2
Cover Story
Perfecting the Image
Listen to this article:
The AI program Midjourney can generate all kinds of images, but it famously struggles with the human hand. Fingers may be missing or added, fingernails absent, and unnatural poses struck. The problem, says Professor Han Kai, Assistant Professor in the School of Computing and Data Science and Director of the Visual AI Lab, is in the programming.
A massive amount of data is fed into AI-powered image generators to train their billions of parameters, but the processes by which they produce content remain a black box – not only to outsiders but, it would seem, to the model developers themselves.
“When existing commercial models were initially trained, these problems weren’t considered, or the right parameters were not injected into them in terms of following geometric, physics and other basic laws,” he said.
Professor Han and his team are working to address that. “In our Lab, we are trying to build models that can actually understand and reconstruct our open world. We want to have a principled understanding of how our models work so they are not just making a random guess.”
His work covers four overlapping areas: understanding and creating images of things, even if the model has never seen them before; creating 3D content; advancing the use of generative AI in image creation; and developing the capacities of existing foundational models.
Open-world training
In the first area, Professor Han and his team have developed open-world learning techniques in which the model independently discovers and correctly applies new concepts to identify categories of objects that it has not seen before. For instance, if trained on dog and cat images, it can separate birds as their own distinct category.
This is unlike the closed-world training of most other models, which only recognise objects in categories they have trained on. These models also struggle with streams of images and with varying representations, for instance, failing to understand that paintings or emojis of cats are still cat images.
In open-world learning, the model still learns from pre-labelled data but then transfers that knowledge to newly encountered data. In the example of cats and dogs, it knows they have four legs, so it recognises that two-legged birds may be their own category.
The model is also being trained to admit when it cannot recognise an image outside its training distribution. This is called open set recognition, a subset of open-world learning, and it is important for averting the mislabelling of images – imagine an autonomous vehicle that receives mislabelled information about roadworks or people on the street. Even slight distortions of images can mess with the predictions of an over-confident model.
“The worst scenario is when a model has very high confidence in its predictions because if it encounters something outside its learning, it may confidently misclassify it,” Professor Han said. Better to make conservative predictions than wrong ones.
Open source aims
His second area of investigation is in reconstructing objects and scenes in 3D or 4D from text and single images. This is tricky work and closely related to his third area of study, generative AI in image creation. While some private firms already provide text-to-3D content generation, such as Common Sense Machines, universities and other users have to pay to use them and users often need special expertise. Professor Han is therefore trying a different approach.
His aim is to produce high-quality images on a limited budget and make this open source for anyone to use with minimal training. For instance, users could extract an object from one image and place it in a different environment simply by typing in some text. The model could find application in things like animation, gaming, education and even creating one’s own avatar to upload to TikTok, although that is still some way in the future.
Professor Han is also working with existing models – his fourth area of focus – to see if they can perform tasks other than those they were designed for, such as matching images to text. He and his team have been testing the capabilities of various models and found Google Gemini 1.5 Pro, GPT 4.0 and Claude’s Sonnet all performed ‘reasonably well’, but still had improvements they could make.
Another ongoing study has set up different AI models to play games against each other, such as chess and Pong, in order to see if their decision-making processes can be discerned.
Professor Han said his Lab’s findings could ultimately have applications in autonomous driving, drug discovery, medical image analysis, surveillance, as well as gaming and entertainment – anything that relies on images. “We are trying to figure out what the key problems are and develop solutions. There are still a lot of problems to address in this space,” he said.
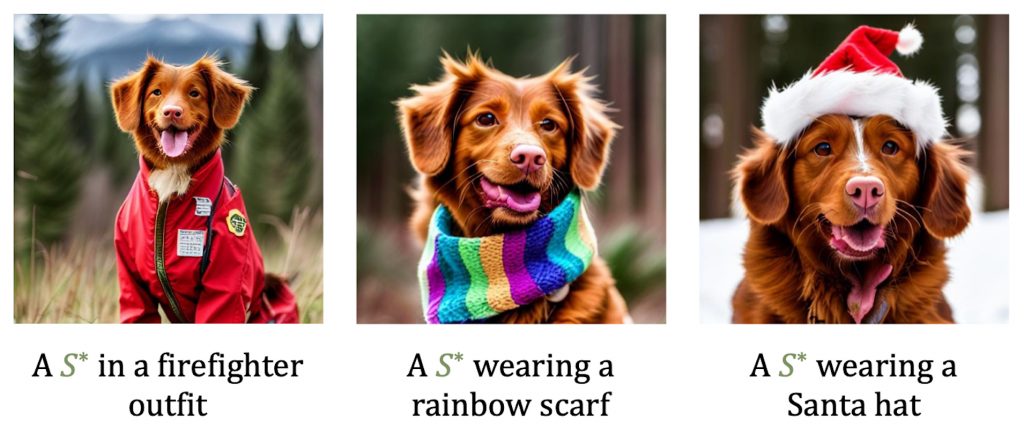
The text-to-image generation model developed by Professor Han and his team is capable of learning a visual concept from a set of images, encoding it as a visual token ‘S*’, and then regenerating it in diverse, previously unseen scenarios.
The worst scenario is when a model has very high confidence in its predictions because if it encounters something outside its learning, it may confidently misclassify it.
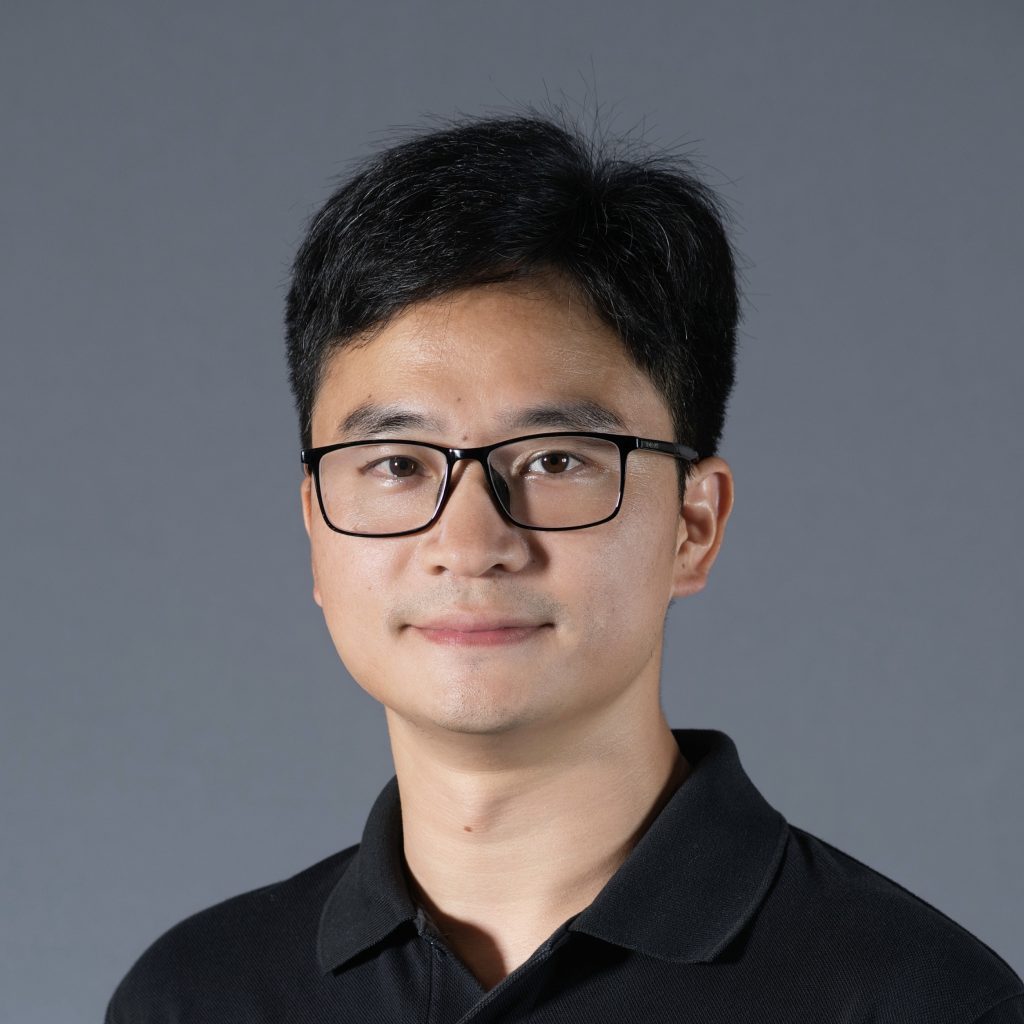
Professor Han Kai