May 2025 | Volume 26 No. 2
Cover Story
Smart Eyes on the Street
Listen to this article:
Urban planning that fails to account for human perceptions often results in environments that disadvantage vulnerable population groups. For instance, if you stand on a tree-lined urban street at lunchtime on a sunny day, the greenery will be a welcome canopy of shade. But stand at that same spot at 11pm and the shadowy trees feel altogether more sinister, especially if you are a woman.
Reconciling such discrepancies is a challenge for planners, who typically have access to mainly day-time views from sources such as Google Maps. Professor Qiu Waishan, Assistant Professor in the Faculty of Architecture, is working to address that and other urban planning issues through computer vision tools that bring human perspectives into consideration.
“People’s perception of urban spaces has been overlooked in terms of how this affects individual decisions and contributes to a city’s economic prosperity. For instance, it can affect whether someone wants to buy a house or rent a workspace there or go out at night. If you want to energise the urban space, then how people perceive that destination is important,” he said.
Until recently, it was nearly impossible to measure and incorporate people’s perspectives and behaviours. Early attempts by researchers involved manually tracking individuals on the street, a time-consuming process that, without supportive technologies, limited observations to narrow areas in single cities.
But about six years ago, when Professor Qiu was a researcher at the Senseable City Lab of the Massachusetts Institute of Technology, he realised that computer vision programs could perform those observations rapidly and across multiple places at once.
Windows, human scale and variety
He set up a website to get baseline data by asking people for feedback on street pictures, such as whether a scene made them feel safe or was aesthetically appealing. That input was used to create labels of street features and establish a training dataset to predict how people might perceive a given city street and how those perceptions might affect behaviour.
The model was then used in real life. For instance, a study on housing purchases and rentals in Shanghai showed that quite apart from the usual external factors of location and accessibility to parks and schools, perceptions of better visual quality as measured by the model coincided with higher housing prices. This effect had not been tested before.
Professor Qiu’s research has also identified factors that provoke positive feelings in a street environment. Transparency of the street’s building façade – essentially how many large windows there are at street level – matters. So does the ‘human scale’ of the street, meaning the ratio of building heights to street width, which is best at a one-to-one ratio. Street complexity is also important, in terms of a variety of trees, signposts, lights, outdoor settings and spots for people to gather.
However, perceptions of visual quality can depend on what a person is doing on that street. A cyclist may prefer lots of trees, while a pedestrian will favour wide sidewalks and places to meet up and socialise.
Professor Qiu’s research also found that while people feel safer when there are others on the street, this is not necessarily correlated with less crime – crimes of opportunity, especially theft, can increase with more people.
Night vision
Moreover, the shadows and coverage provided by trees can affect a sense of personal safety, as the example of women at night illustrates. He is also working to address that issue by applying computer vision and artificial intelligence to predict nighttime urban spaces based on daytime images in his dataset.
“This is ongoing research, so we are still collecting paired day and night street view images, but the results so far are quite amazing. Our aim is to produce a model that can help urban designers and planners around the world to predict their local nighttime environment,” he said.
He is also trying to predict people’s behaviour to inform better planning. Data collected through computer vision can show such things as the kinds of shops that attract more pedestrians and whether special equipment encourages people to exercise. The behavioural data is collected carefully to protect privacy – faces are blurred and the focus is on crowd behaviour, not individuals, he said.
Professor Qiu has also used his model to show how urban visual quality can attract innovators. He compared innovation hubs in London, Boston, Seattle and San Francisco with Shanghai’s Zhangjiang Science City. The other global hubs scored 7.0–7.5 for visual quality, while Shanghai’s uneven and polarised urban development meant some established parts scored 7.5 while others only about 2.0. Along this line, visual consistency is a fourth factor affecting street visual quality, in addition to transparency, human scale and complexity.
“We need to pay attention to homogenising building façades. Every city wants landmarks, but if every building is trying to be the region’s rockstar, it can look very distracting and overwhelming, making people feel anxious,” he added.
If you want to energise the urban space, then how people perceive that destination is important.
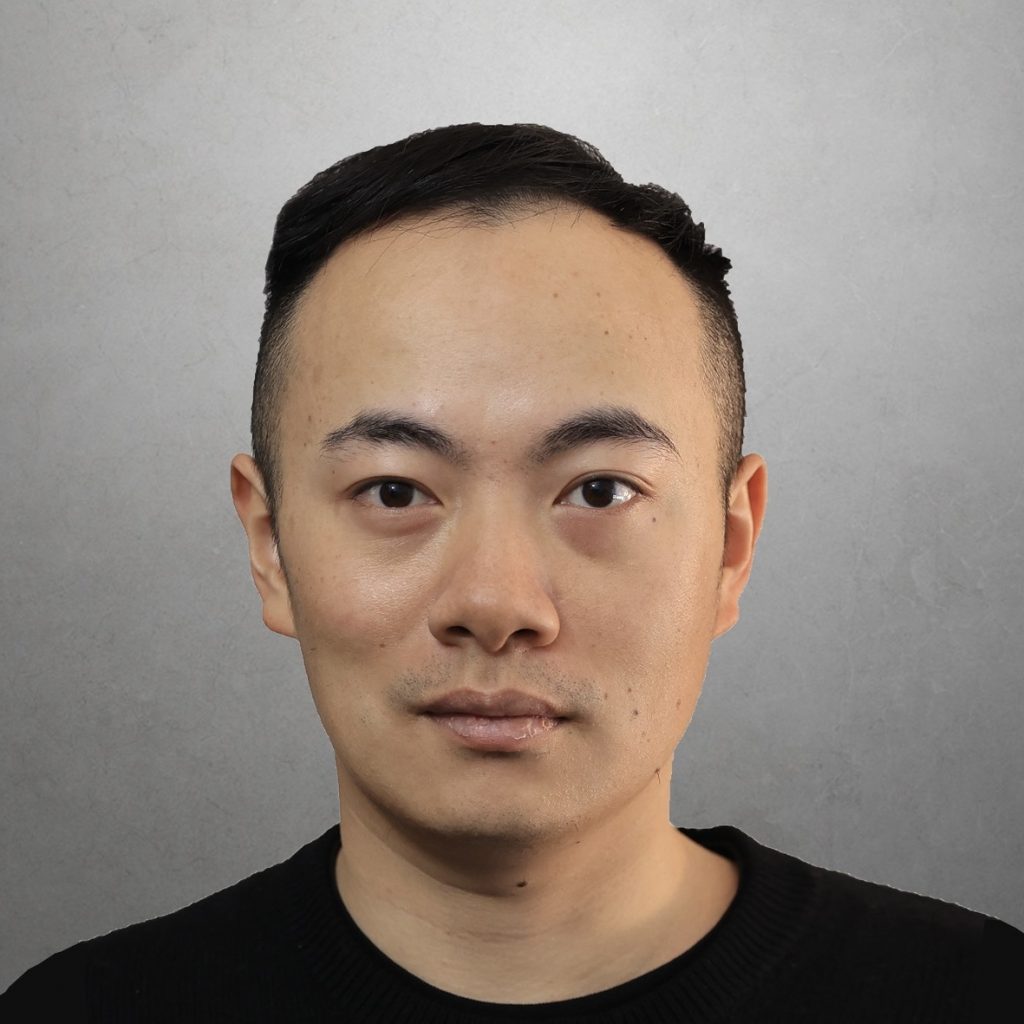
Professor Qiu Waishan